The classifier is the central AI component of the ringface solution. It implements the AI algorithm of face embedding, and the Machine Learning concept of SVM Classification. In essence it offers two methods (fitting and face detection), that we will expose as cloud function.
We will make the necessary changes to this component to lift it to GCP from the docker environment. As the classifier itself is run infrequently, and while it requires significant resources to run the image processing and AI tasks, it is best candidate for another Cloud Function: we can assign it ample hardware, yet it will only incur costs when we actually execute it.
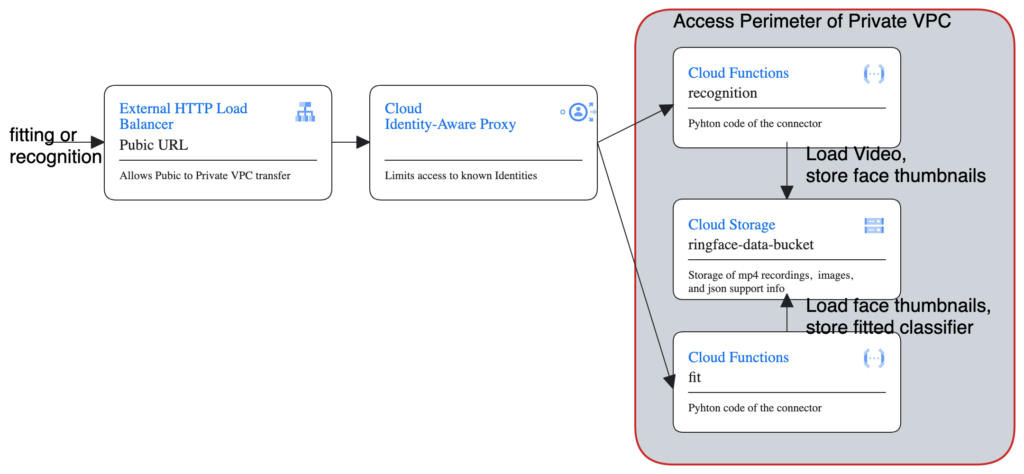
Terraform changes
As we have already introduced the load balancer components in the previous post, the changes here are relatively simple. We need to enhance our URL map to add the paths for the new functions.
resource "google_compute_url_map" "ringface_url_map" {
name = "${var.project_id}-url-map"
default_service = google_compute_backend_service.ringface_backend_service.id
host_rule {
hosts = ["*"]
path_matcher = "functions"
}
path_matcher {
name = "functions"
default_service = google_compute_backend_service.ringface_backend_service.id
path_rule {
paths = ["/download"]
service = google_compute_backend_service.download_ring_video_neg_backend_service.id
}
path_rule {
paths = ["/fit"]
service = google_compute_backend_service.fit_backend_service.id
}
path_rule {
paths = ["/recognition"]
service = google_compute_backend_service.recognition_backend_service.id
}
}
}
The resulting code is in this GitHub tag.
Image processing
Changes on the Python codebase are more profound. We will need to replace all local file system access with Cloud Storage access, something that is not really supported well in all the image processing libraries. The strategy is to use an IOBuffer object to provide a filelike object where possible, and to download to /tmp a copy of the Cloud Storage Object where direct memory buffering is not available. The latter is the case for the opencv library, used for loading the video sequence.
def filelike_for_read(filename):
logging.debug(f"Will expose {BUCKET_NAME} and {filename} as file")
data = blob(filename).download_as_bytes()
logging.debug("Blob data loaded")
return io.BytesIO(data)
def tmpfile_for_read(filename):
tmp_file = f"/tmp/{os.path.basename(filename)}"
logging.debug(f"Will expose {BUCKET_NAME} and {filename} as {tmp_file}")
data = blob(filename).download_to_filename(tmp_file)
return tmp_file
As image processing is a rather cpu and memory intensive task, and it is ridiculously parallel, we will deploy the function on top of a 4 CPU VM (line 10).
gcloud functions deploy recognition \
--gen2 \
--project $PROJECT \
--service-account classifier-sa@$PROJECT.iam.gserviceaccount.com \
--runtime python310 \
--source=. \
--entry-point=recognition \
--trigger-http \
--memory 4GiB \
--cpu=4 \
--region=europe-west3 \
--allow-unauthenticated \
--ingress-settings internal-and-gclb \
--env-vars-file .env.yaml
To see all the code changes, refer to this GitHub tag.